This post first appeared on Quite A Few Claims on 4/20/2023 at https://quiteafewclaims.substack.com/p/ai-has-entered-the-chat
Blake’s Foreword: I’m excited to have collaborated on this post with Ben Lee from Quite a Few Claims and Paulius Mui, MD from X=Primary Care. Special thanks to Ben who QB’d the entire process and put together the bulk of this piece on clinical AI. I hope you enjoy – it’s a good one!
- Subscribe to Quite a Few Claims
- Subscribe to X=Primary Care
- If you’re new here, subscribe to Hospitalogy
The Era of AI-Enabled Clinical Workflow
By Ben Lee
Yeah, it’s another AI post in a sea of them. First of all, a self-deprecative disclaimer:
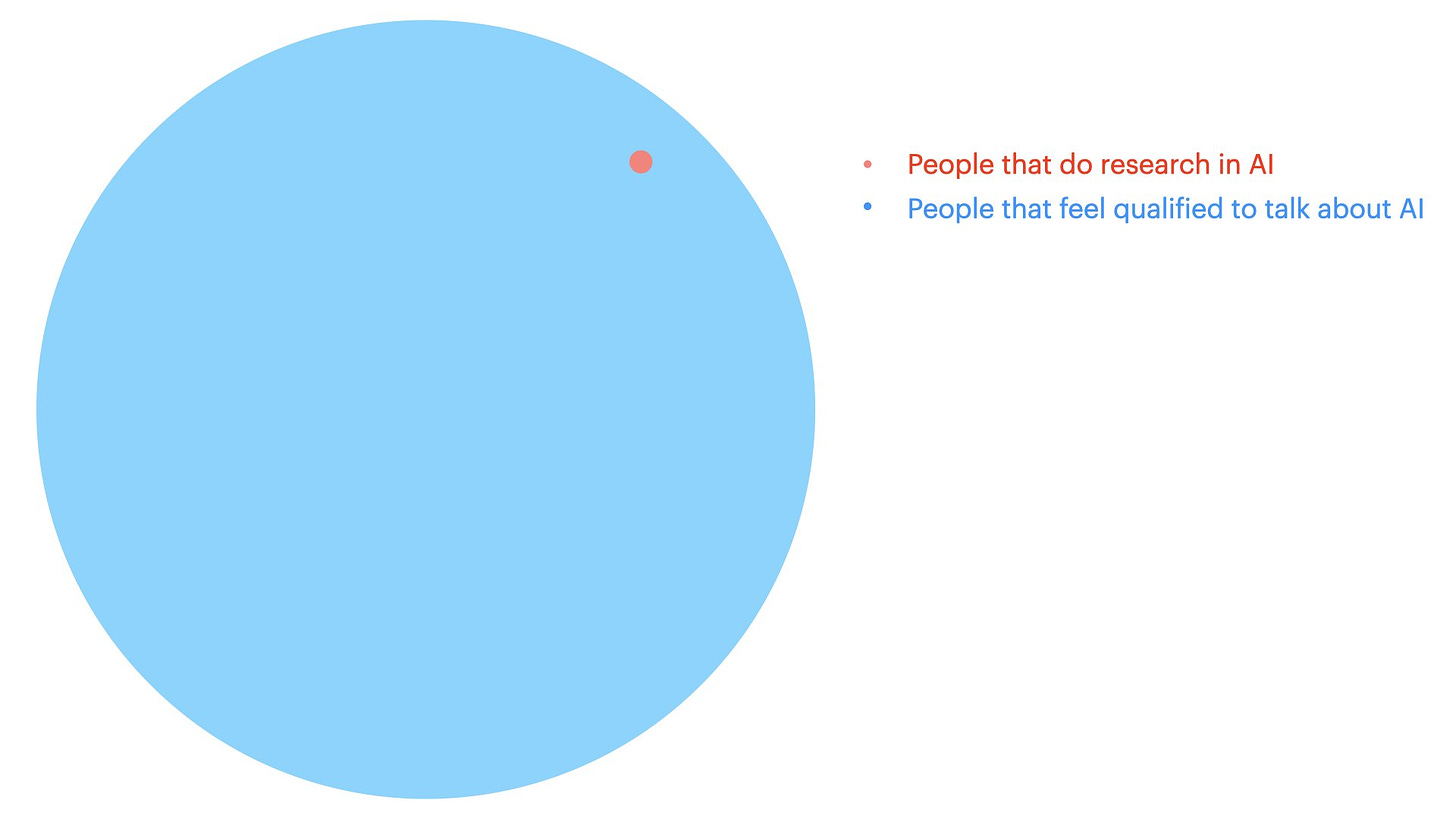
Below, we cover:
- Context on what’s led up to the current AI zeitgeist
- Opportunity spaces for AI applications in clinical workflow where players are converging, and why
- Segmenting the current space and the competition dynamics between players
- Anticipating how new players can make space for themselves in the space
- A clinician’s perspective on these happenings, by Paulius Mui
- A look at the recent financial and commercial activity in the space, by Blake Madden
This post is hefty – thanks for reading in advance 🙂
Broad AI Context
In 2017, the Google Brain team’s landmark paper Attention Is All You Need introduced the Transformer architecture, which drastically improved performance and quality in building large language models (LLMs). What followed was a convergence in approach across ML / AI research, resulting in composability of ideas and compounding of improvements. 2020 saw the OpenAI team publish Scaling Laws for Neural Language Models which led to the emergence of the Scaling Hypothesis – TL;DR: pump model size, data, and compute and you’ll see increasingly sophisticated behavior in AI models. Then of course, a small “research preview” called ChatGPT surfaced the capabilities and potential of LLMs through a consumer-facing interface, and became the fastest growing consumer app of all time (100M MAUs in just two months!). Here’s a fantastic primer on most of the concepts behind the tech.
The resulting Cambrian explosion of AI innovation has occurred at a breakneck pace – so much so that, a few days ago, many notable figures across many disciplines signed an open letter to pause large scale AI experimentation for six months. Everything is happening everywhere, all at once.
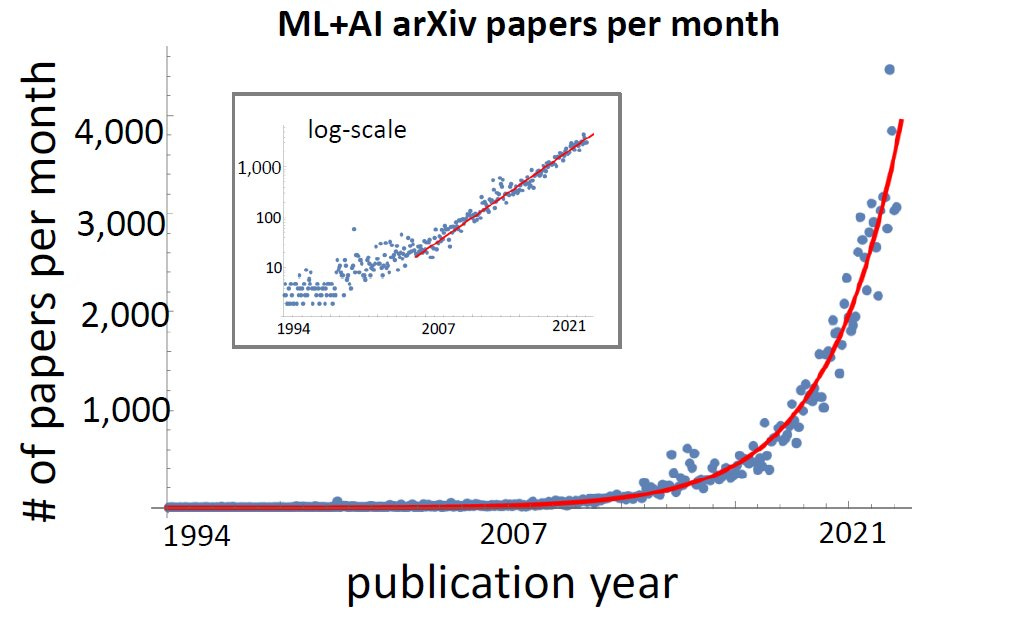
Although the healthcare industry has been burned in years past by AI overpromise and the low use case / complexity ratio of task-specific models, the current moment feels like an inflection point for the industry as well, specifically due to the massive leap in performance. For one example: LLMs aren’t just passing the USMLE, they’re crushing it (GPT-4: 86.65%; Google’s Med-PaLM 2: 85% – both roughly the 90th percentile). Commonly stated concerns include hallucinations, equity & bias, and automation bias among others (interesting Chrissy Farr thread below).
My (Ben’s) take: the degree of attention and pace of innovation in the space will eventually render these concerns largely obsolete, although it is certainly likely that new ones will rise. For instance: inaccuracies in mathematical calculations were among the most commonly observed hallucinations – OpenAI’s introduction of third party plug-ins, and Wolfram-Alpha’s development of a plug-in has already dramatically reduced such mathematical hallucinations.
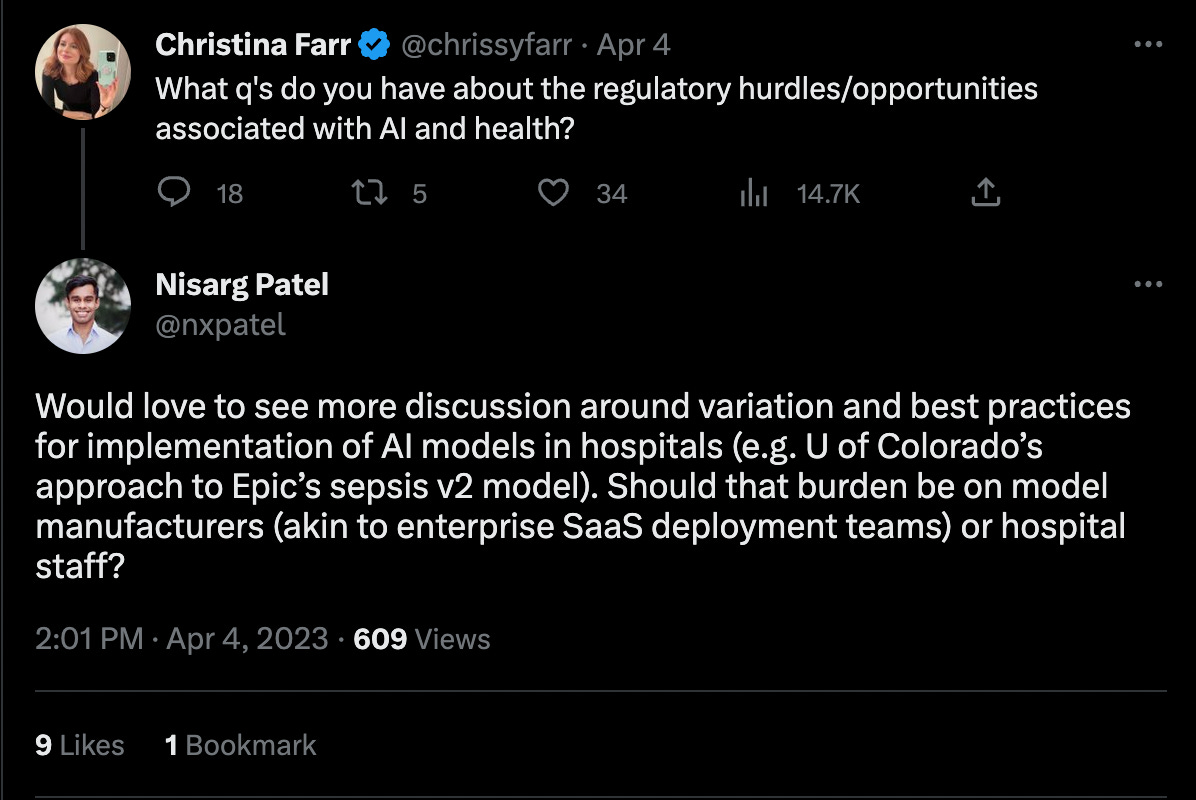
And the industry is responding accordingly. New healthcare-specific thoughts and papers (like this one!). Rapid fire Twitter musings by all who dare to comment. AI-focused roles from prompt engineers to the emergence of the Chief AI Officer in health systems – the latter certainly implies a sense of urgency in health system IT departments. The road to implementation & end user adoption at health systems is crowded with provider app vendors scraping by awaiting CISO legal / security review and integration – the mentioned trend might provide a zipline to the top for some chosen few.
Clinical Workflow in the Age of AI
The focus has predominantly landed on solving the increasingly burning problem of provider supply shortage – this is also where we’ll focus for the bulk of this post. Some more applications here by Eric Topol for your reference.
Here’s a quick(est) recap. Demand for care is slowly growing and will likely accelerate due to many factors including a growing, aging population and likelihood of higher patient acuity. This demand is far outpacing supply – the provider shortage is expected to be at ~57000 FTEs in 2025, and growing year by year.
As the shortage exacerbates, increasing pressure is put on providers to deliver more care with the same resources. Compounding this further are the burdens of administrative tasks placed on the provider – many related to the much-maligned UX of EMRs. This has resulted in high levels of burnout, driving physicians to retire or pivot roles/industries, and – you guessed it – increasing shortage.
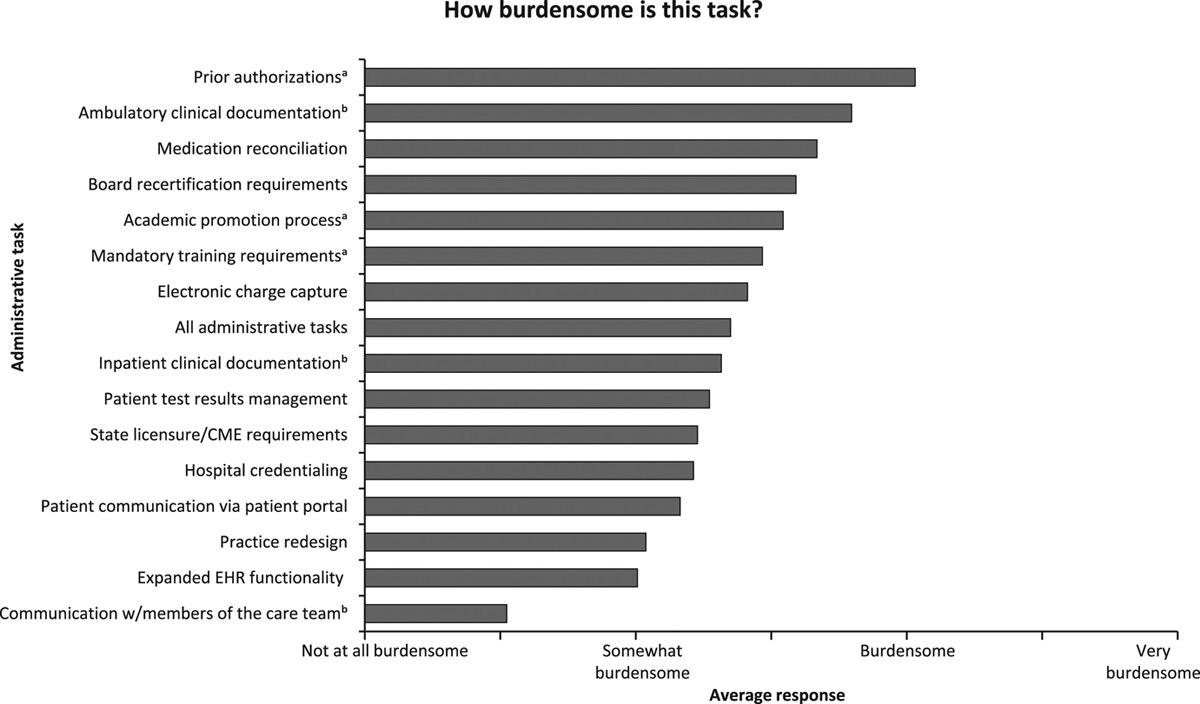
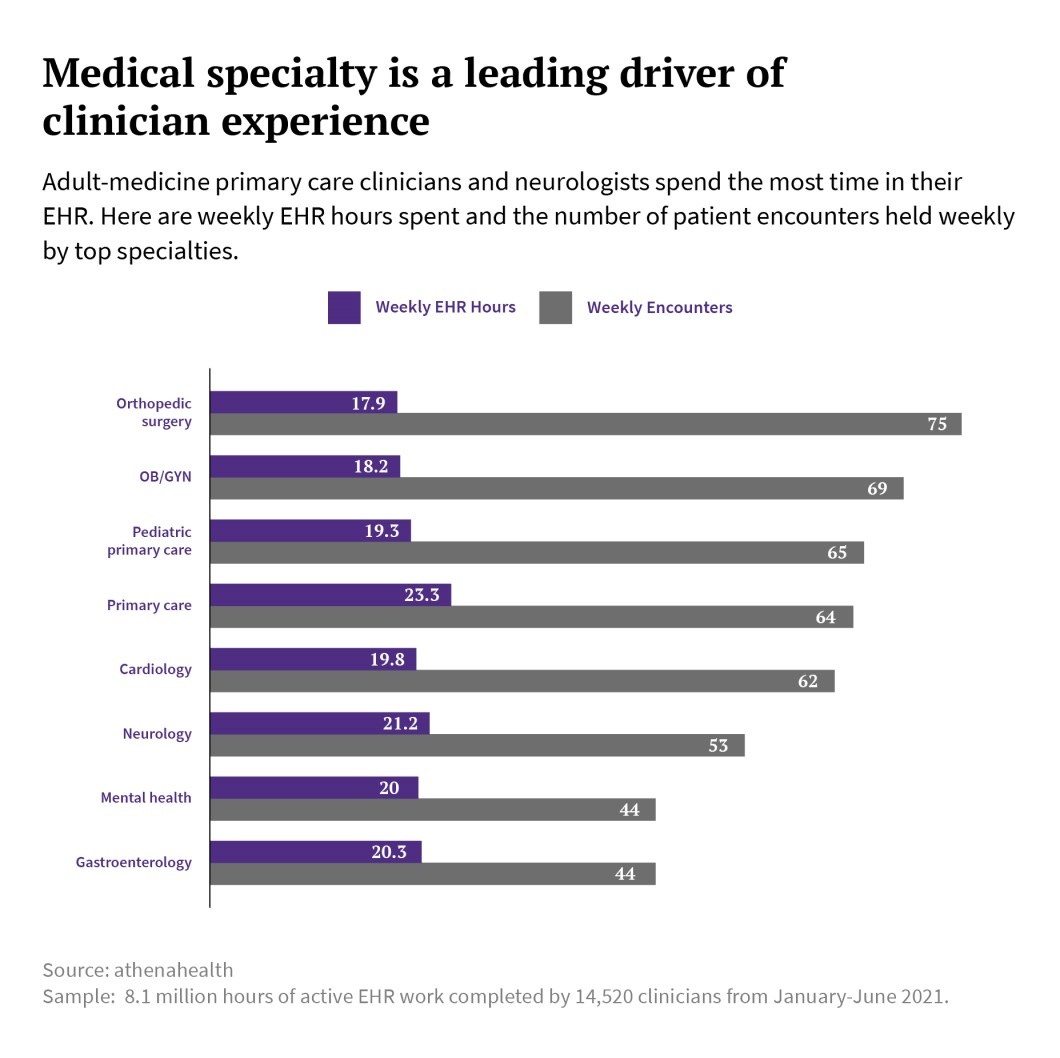
In particular, the documentation and prior authorization spaces have received attention from builders, not only due to the severity of their burden on clinicians, but also their positions as key junctions connecting workflows in clinical operations, patient data, and reimbursement. The past ~decade saw a variety of approaches, ranging from RPA to integration-heavy sidecar apps to net new EMRs; you could count AI-enhanced products among these, but all such products required humans-in-the-loop to close the last mile of performance. (More on this later)
The recent leap in performance and quality of generative AI has resulted in renewed attention from many that are drawing the dots between the qualities of these tasks – time intensive, manual, delegable, content heavy – and the strengths of LLMs.
Join the thousands of healthcare professionals who read Hospitalogy
Subscribe to get expert analysis on healthcare M&A, strategy, finance, and markets.
No spam. Unsubscribe any time.
Who’s getting involved?
What’s interesting about what’s happening is the democratized access and capabilities for personas across varying degrees of complexity and healthcare involvement, including:
- Care team end users using ChatGPT as a means to alleviate their current workflow pain points
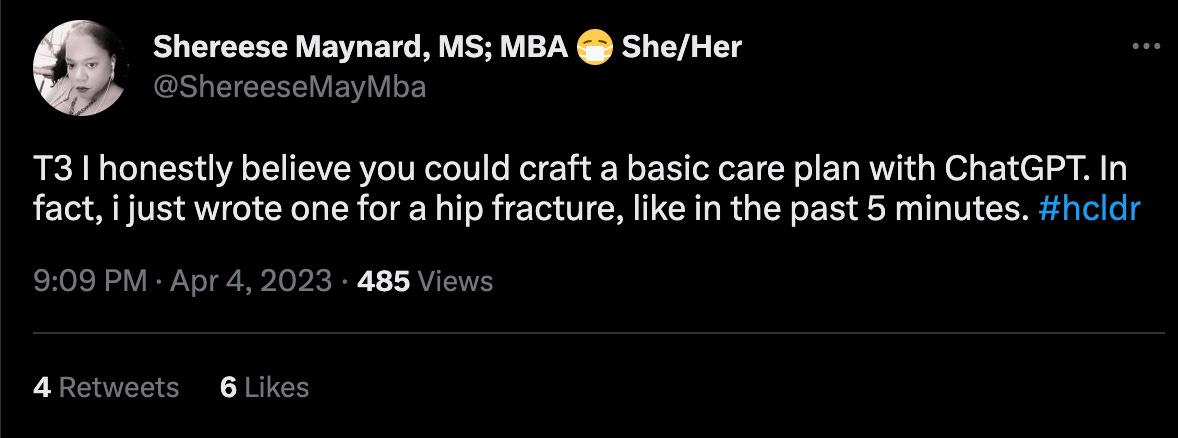
- Care delivery organizations buying AI solutions from vendors creating their own AI-enabled tools for the workflows of their in-house care teams (Curai, which has been AI-focused since inception; tech-enabled orgs – quote below from VP of Prod at Oak Street Health)
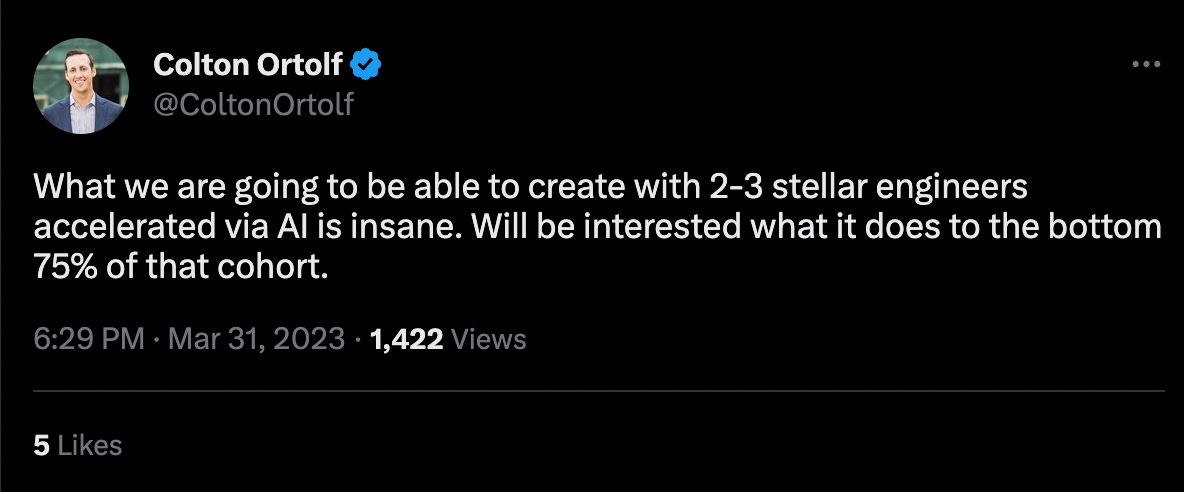
- Net new AI-native SaaS organizations building for care delivery organizations (Abridge, Ambience, Nabla, Ethermed, Glass Health and Fairway from the latest YC batch, more orgs here)
- Incumbent healthcare SaaS organizations transitioning to selling AI-enabled feature sets (Epic, Nuance, Augmedix, Doximity)
- Industry-agnostic, large-scale orgs pouring $xxB in healthcare ventures (Microsoft, OpenAI, Google, etc.)
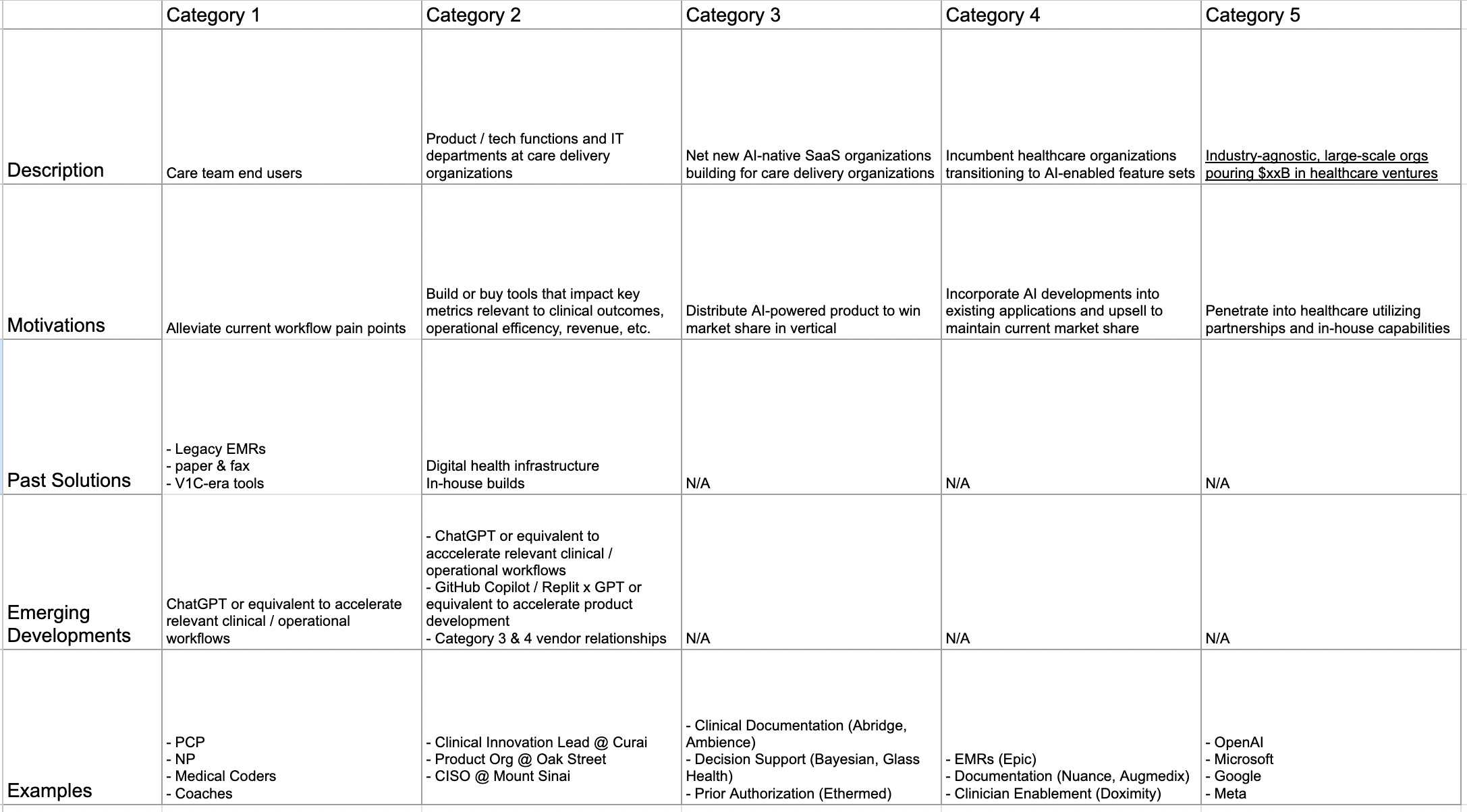
History and Current Dynamics
The documentation space (the most crowded one) has intriguing dynamics that may be translatable to other spaces. Let’s focus first on the healthcare-focused SaaS organizations, which would be categories 3 and 4.
Category 4 contains the more veteran players (namely Nuance, Augmedix; 3M’s M-Modal, also Suki) that have weathered the tumultuous path of healthcare industry distribution and its many challenges – long sales and implementation cycles, bespoke integrations, etc. – with services-heavy models. Serving as primary trailblazers in commercializing novel technologies (in this case, applying ASR + NLP / LLMs towards ambient documentation), these companies often augmented the premature capabilities of their models with humans-in-the-loop – typically cheaper talent such as offshore scribes, medical students.
These hybrid (human + AI) models proved superior to status quo; training and retraining human scribes took time, while dictation software and other AI-enabled competitors proved actually additive to clinician burden by requiring edits. Yet, hybrid models also offset the production cost and scalability benefits of AI, restricting distribution to larger organizations that can afford them.
The holy grail, however, remained full automation (in documentation – real-time, structured output that accounts for the full breadth of care settings) and the venture scale margins that come with it. With continual research elevating AI capabilities, new entrants (Category 3 – Abridge, Ambience) have surfaced who promise just that: near-to-full automation of administrative burdens and restoration of clinician time. A bearish perspective to take here would be to view valuable doctors and clinician end-users as the humans-in-the-loop (as opposed to the cheaper talent deployed by Category 4).
However, the most compelling among Category 3 do boast in-house high pedigree AI/ML talent and data partnerships that enable model fine-tuning (in pursuit of closing the last mile of quality). If we are to assume that the quality of models/products have reached (or will reach) sufficient enablement of full automation, Category 3 now faces the uphill battle of healthcare distribution – an arena where Category 4 orgs sit as advantaged incumbents.
Furthermore, many in Category 3 are beginning to strategize around second order implications of LLMs in healthcare. If the first wave of AI tools eliminates the negatives of clinician workflow, how does one then add positives and empower providers? What are the compelling ways one might compress downstream tasks or utilize real-time data generation towards care advancements? Pulling from the popular example of Github Copilot and its abilities to enhance developer productivity (rather remarkably, as the below chart shows), players here are positioning themselves towards this direction with words like “copilot”, “superpower” in their copy.
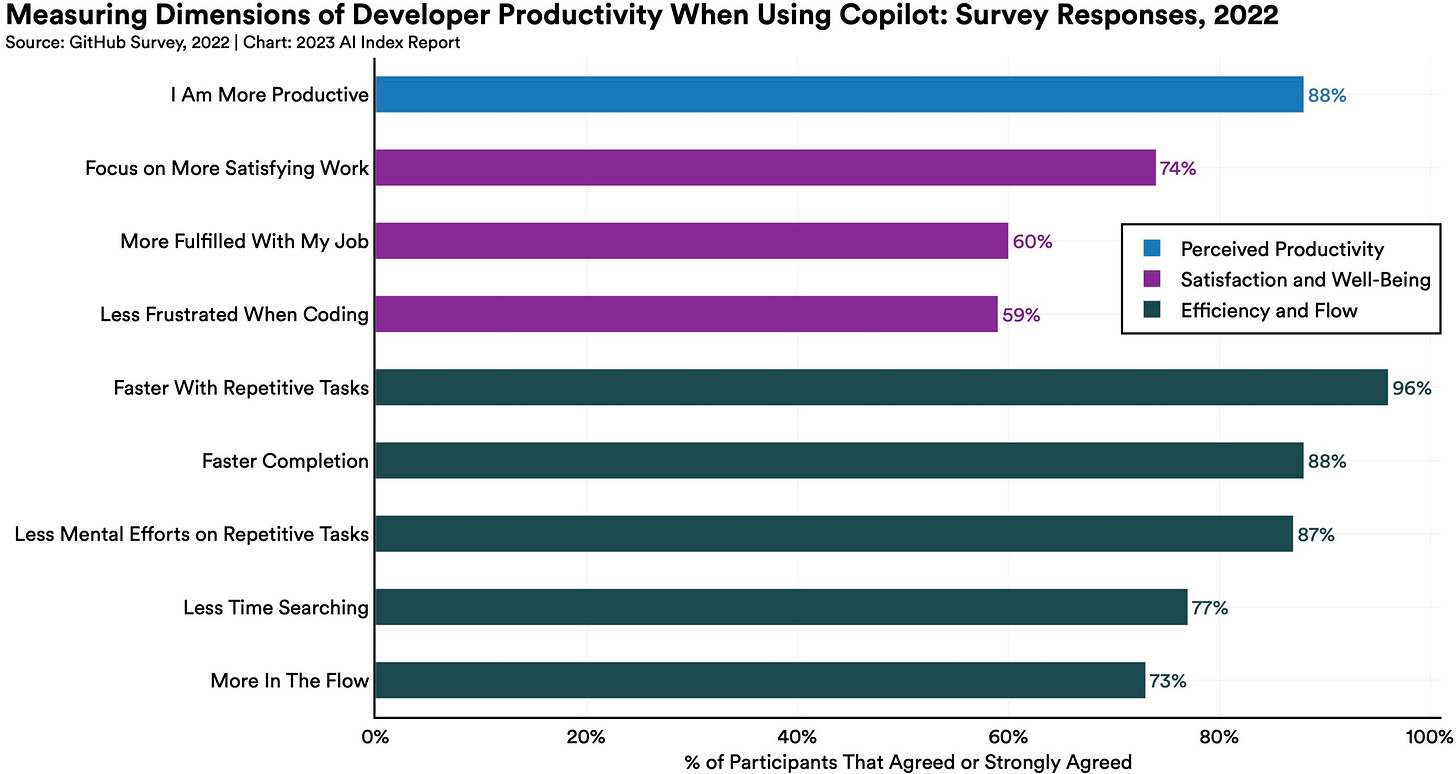
As there is yet to be an establishment of standardized benchmarks, it’s tricky to compare existing products beyond anecdotes and marketing language. This will be all the more critical as the space becomes saturated.
Heeding both the general capabilities & hype surrounding recent AI developments and the increasing threat of newer Category 3 organizations, Category 3 veterans have returned back into public eye in recent years, with particular types of activity:
- Collaborating with Category 5 industry-agnostic tech behemoths through partnerships / acquisitions as a means for augmentation of AI capabilities and channel partnerships towards logo collection (Nuance acquired by Microsoft; Augmedix with Google, 3M collaborating with Amazon)
- Announcements to release fully automated / generative AI solutions later this year (Nuance injecting GPT-4 into their ambient documentation product DAX Express; Augmedix piloting Augmedix Go, Suki announcement)
How will this play out?
Let’s take a quick step back. AI innovations are happening in almost every sector and function, including software development. Tools like the aforementioned Github Copilot to code creation enablers (Insane open source tool here – AutoGPT) are drastically driving down the marginal cost of building software. Applying this to the industry, small teams (or even one person) can build projects solving for previously labor-intensive healthcare problems in a fraction of the time.
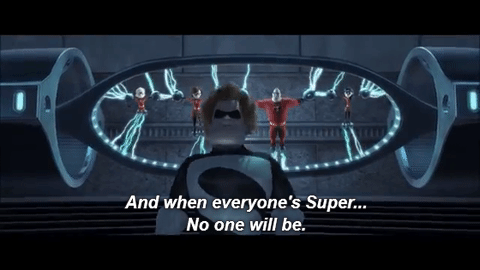
It’s fair to cite history in being bearish towards ML use cases in healthcare reaching real life adoption, with many failing to bridge the implementation gap. The required time & costs of change management during deployment have battered many a challenger.
Still, here are examples of demos for medical coding automation built by one person – though likely currently far from suitable for enterprise at scale, is it that much of a stretch to think that something of that grade, for many AI-appropriate needs, will arise within the coming months given the pace of innovation?
The compounding ease with which one can inject AI into workflows and build applications means that the time window for startups (and Category 3 players, documentation or otherwise) to take advantage of some of the classic items in their favor – speed-to-market vs. Innovator’s Dilemma, etc., becomes compressed.
If one person can stand up a demo in less than a month, Category 4 incumbents for any workflow vertical should relatively quickly establish a new status quo of AI-enabled experience through deployment at scale across their sites without need to grind away at health system distribution and implementation, easily the heaviest bottleneck. Yet, point solutions in Category 4 themselves will be fearful of the foundational platforms and systems of record (namely Epic and its GPT-4 partnership). Related to generative AI for Epic in particular, the EHR giant is building generative AI tools on Microsoft Azure’s Nebula – Azure’s public cloud offering. Organizations can connect to Nebula regardless of whether or not they’re on-prem, using Epic-hosting, or using a public cloud for their Epic instance. Epic has been deploying multiple gen AI functions across clinical predictive models, geolocation services, and an SMS gateway on Nebula – consequently, scaling is less of a concern here.
And that doesn’t even begin to mention all the use cases that simply OpenAI + industry plug-ins eventually can and will sufficiently cover.
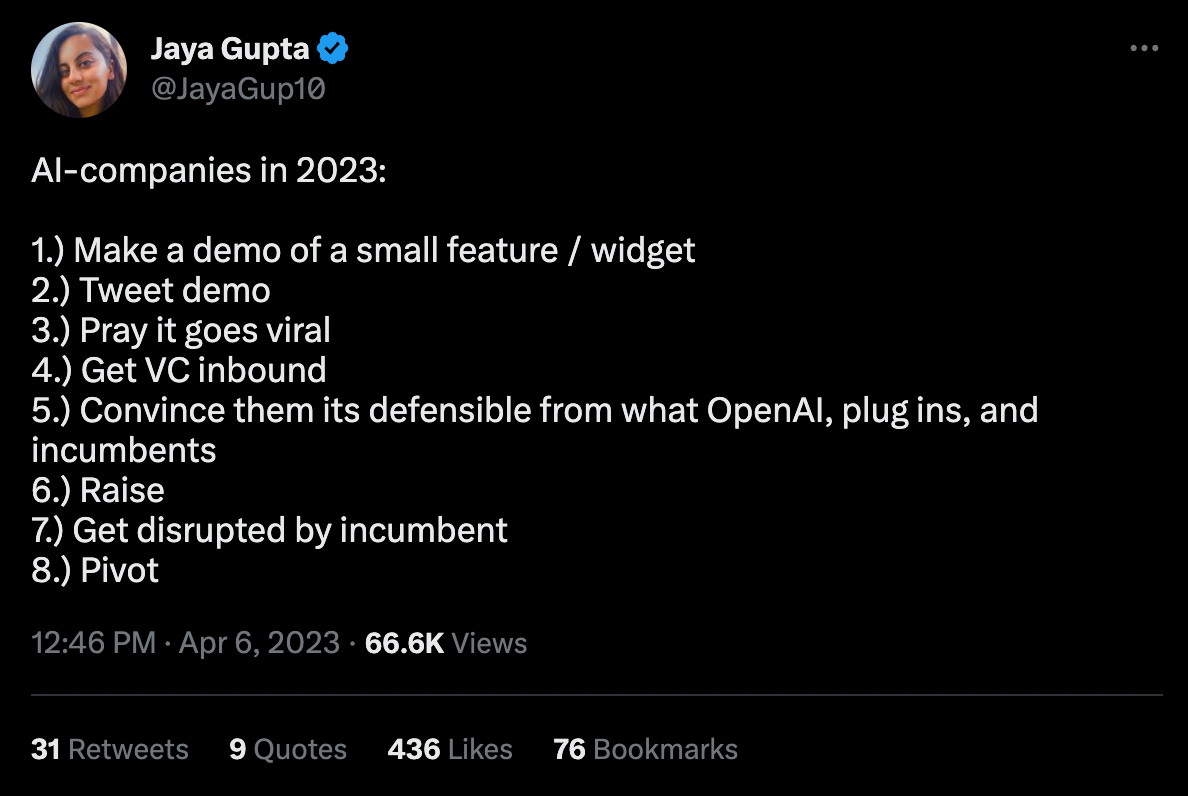
This begs the question: in this arena, what will the organizations that win look like? Do we chalk up the victory to incumbents and OpenAI and call it a day?
In the nearest term, well-known dynamics will persist. Many care delivery organizations (Category 2), in particular the larger ones with thin margins and strapped for resources, will be driven to address clinician shortage needs by partnering with vendors providing near-term ROI.
For even the small-to-medium care delivery orgs capable of experimenting with AI workflow injection, most will gravitate towards their primary business focuses in a capital-strapped environment, and perform risk-averse AI experiments at most as opposed to re-evaluate their operating model for AI-enabled efficiency improvements.
As such, the TAM of provider org prospects will remain stable, and such prospects will drive towards vendors (3, 4, maybe 5). The battle here will be between how quickly AI-Native Category 3 players can distribute and implement vs how quickly Category 4 can build a “good enough” solution and upsell existing relationships. Point to Category 4 incumbents.
A note on data moats
Currently, proprietary data is commonly cited as a moat, which provides a massive advantage towards incumbents. I’m not yet convinced that it’s unconquerable (someone please correct me) in the longest term. Below are some early observations:
- Outputs from a model can be used to train other’s (including competitors)
- Fine-tuning seems to cost less and require less data
- There’s at least some likelihood that future GPT-X models outperform specialized models
- Increasing data interoperability and data structuring ability in healthcare
Naturally, possession of such data provides accelerated speed-to-market for incumbents in the near term, which buys time for further advancements. Further, there’s still instances where proprietary data will be paramount (biotech for one). However, especially for use cases where a qualified individual (like clinicians) can verify and edit output (and such flow can even be a trust-building component), there might be diminishing marginal returns.
Differentiation
When Category 3 new builders might begin to take advantage is in the medium-term, with a fundamental and annoyingly cliche answer: “good product execution”. How good and fast are you at uniquely identifying the right problems for your customer, and subsequently the right things to build?
Some thoughts here on how AI-native organizations can start to gain market share:
- Agility in idea generation and constant delivery of innovative “AI-native” workflows allow the steady march towards overall differentiation. My (Ben’s) hypothesis: all incumbents will be able to relatively easily develop and deploy “point AI-feature sets”; however, AI-native organizations can build interlinked AI-enabled workflows towards a cohesive AI-enabled product ecosystem. This will be more difficult to replicate and provide an overall more effective experience than incumbents embedding AI into legacy applications. Examples:
- Point feature sets
- Real time patient data value unlock
- AI-induced synthesis of clinical corpus
- Interlinked workflows
- AI-induced inter- and intra- product task automation from AI-generated output (Agents?)
- Point feature sets
- Workflows that work in harmony with existing healthcare systems and processes will be a no brainer. Layering this with workflows/interfaces that can transcend the traditional lock-in of healthcare systems enables flexibility in UX and hopefully sticky end user adoption. Many of Category 3’s gravitation towards browser-based or app-based workflows aligns with this concept.
- On the themes of end users and interlinked workflows, Category 3 players might find it interesting to optimize for product network effects to accelerate sticky end user distribution. An example might be collaborative feature sets that enable care teams to deliver better work more quickly when more users use the product together.
- Despite my earlier disparagement of data as a moat in this realm, constructing data network effects to continually extend data moat could be a compelling way to stay on top of the curve. This often means finding methods to continually elicit contribution from end users, which does seem to interlace well with RLHF (the most popular fine tuning method).
- There’s increasing optimism around product-led growth (PLG) for healthcare SaaS. It’s critical to note PHI concerns (great thread by Brendan Keeler here) for most use cases, but DocsGPT by Doximity and Glass Health achieving some virality across clinician circles show proof of concept – there’s something here. Further, open source efforts are surfacing quality LLMs that can be self-hosted and fine-tuned and enable circumvention of cross-organizational data transactions.
Of course, all this needs to be combined with effective change management especially for larger provider organizations.
From Rohan D’Souza (former CPO at Olive): “One idea might be to create experimentation models within systems to go through full implementations with stakeholder commitment. This will require budget to hold both customer and vendor/partner accountable with a nod to full scale deployment assuming the experiment succeeds. Without this, all we will hear is more AI amazingness in press releases with no actual deployments.”
Long term, constant product execution across the above thoughts will establish 1) brand equity and 2) true end user adoption that translates to an enterprise stickiness surpassing contract-induced “stickiness”. There’s some parallels here to Figma using a new paradigm (browser-based applications) to displace incumbent applications; I think this will be a compelling model for AI-enabled clinical workflow SaaS orgs to take after. Bessemer predicts that AI-Native companies can reach $1B ARR 50% faster than cloud counterparts – in healthcare SaaS, the above outlined thoughts might provide one such path.
Value Shifts and Parting Notes
Lastly, the eventual ubiquity of high-powered LLMs has us look to Clayton Christensen’s The Law of Conservation of Attractive Profits: “The law states that when modularity and commoditization cause attractive profits to disappear at one stage in the value chain, the opportunity to earn attractive profits with proprietary products will usually emerge at an adjacent stage.” If AI deployments achieve massive scale and many clinical workflow tools become commoditized, where then does value materialize in such a case?
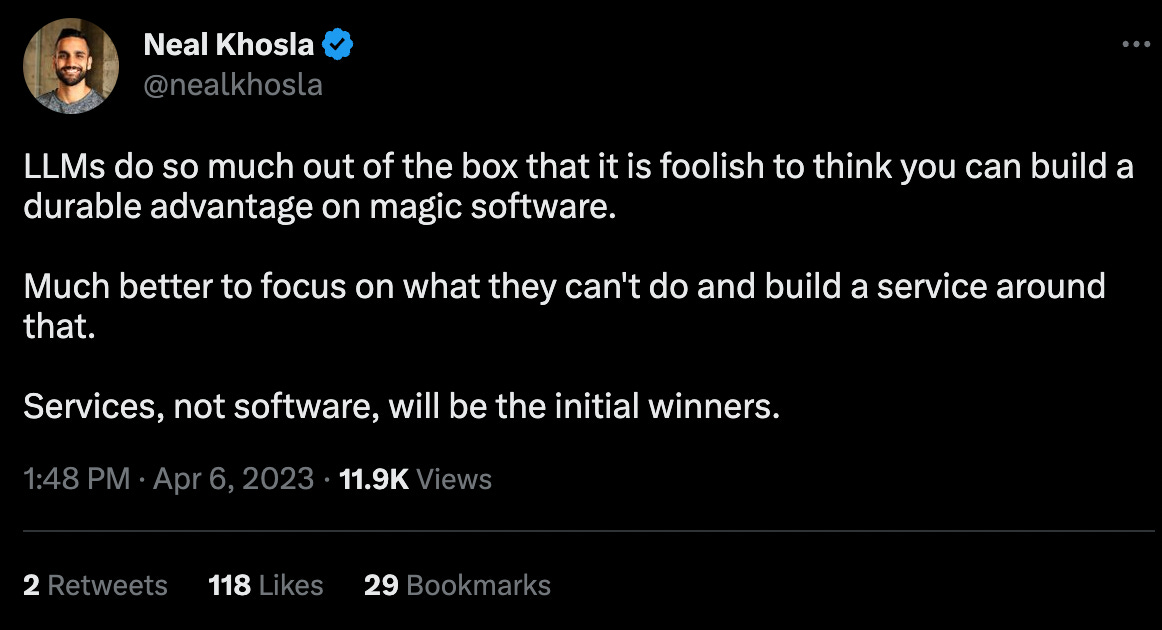
Taking to the law, some early hypotheses to where value might accrue:
- Applications that enable data unlock for any care delivery organization that seeks in-house AI deployment – ScienceIO, Truveta
- “Verticalized” care delivery organizations that embed AI in their life blood across operational & clinical steps to reduce overhead + increase value, competing in selling care delivery as opposed to selling AI – Curai seems to be AI-native and is intriguing to observe from this lens.
- Enablement models (a la Passion Economy) that address administrative pain points to create and aggregate outsized capture of patient value – AI-enabled MSOs / ACOs?
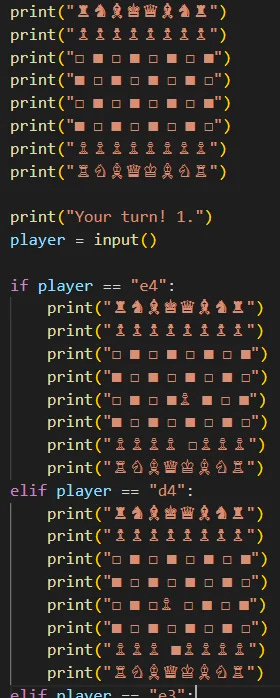
If it isn’t evident through all of the above, I personally am tremendously excited about what’s happening and what’s to come. I’d love to chat if you are as well (or even if you’re a skeptic!) – feel free to drop me a note at [email protected]. Special thanks to Rohan D’Souza, Samy Danesh, Samir Unni, Patrick van Nieuwenhuizen, Rik Renard, Ian Shakil, Alex Barbet, and many others for insights, perspectives, etc. on this post (not endorsements!). And of course, thanks in particular to Paulius and Blake 🙂
A Clinician’s Perspective on AI
Prior to the release of ChatGPT, we published a primer on AI in primary care. The developments in AI since then continue to outpace everyone’s ability to keep up with them. For AI to truly disrupt clinical workflows, the advancements in tech need to be coupled with advancements in payment models. For now, AI tools are limited to tweaking components of the imperfect system. Nonetheless, we’re excited for what’s on the horizon.
Bespoke experiences are becoming the status quo. AI intake and triage tools will relieve access bottlenecks for low acuity, high volume needs in personalized fashion. This will redefine the “front door” experience for all stakeholders. Launch of services like Azure Health Bot lowers the barriers to train own AI models from trusted documents and deliver the kind of responses patients would expect from a doctor who knows them well. To get this right, optimizing reinforcement learning from human feedback will be an essential new clinical workflow for care teams. Scaling data annotation will be a huge unmet need and companies like Centaur Labs will become increasingly relevant.
Patient engagement and data analysis will never look the same. Developments like HealthGPT showcase the potential of integrating personal health history with state of the art AI bots. As privacy and accuracy kinks get ironed out over time, insights into strategies for preventive health and chronic disease maintenance will stem from data across all domains of patient lives: financial, behavioral, and clinical. Primary care teams are uniquely positioned to partner with patients in managing such data assets because of the inherent nature of enduring relationships. Surfacing data intelligence will be a much easier lift without the need for the same depth of resources as it will be simply “conversational.”
There is much debate whether AI tools will facilitate health systems in entrenching their power or will it empower private practice clinicians to break away from incumbent tech to innovate? Standing up a tech forward practice from the ground up has never been simpler. When coupled with tools like Ease and expert full stack dev shops like Light-it, independent practices can deploy enterprise-grade tech at the community level.
It’s evident that low-hanging fruit for AI to tackle is the booming clinical documentation market. “Communication is documentation” concept pioneered in virtual primary care will become the new standard in traditional care settings. These tools will shift the roles of clinicians from content creators to content editors. Will this make healthcare more human? Finding signal among documentation noise is already a real pain point with modern EMRs that may be exacerbated during the transition to a fully ambient documentation paradigm. We anticipate that winners in this space will be those who identify the most appropriate use cases for AI augmentation of the documentation experience based on deep clinical expertise. Leaning into the guidance of clinical advisors will be a key differentiator for AI startups because there is a large disconnect between clinicians and builders. Here are some of the companies whose work we’re following in this space.
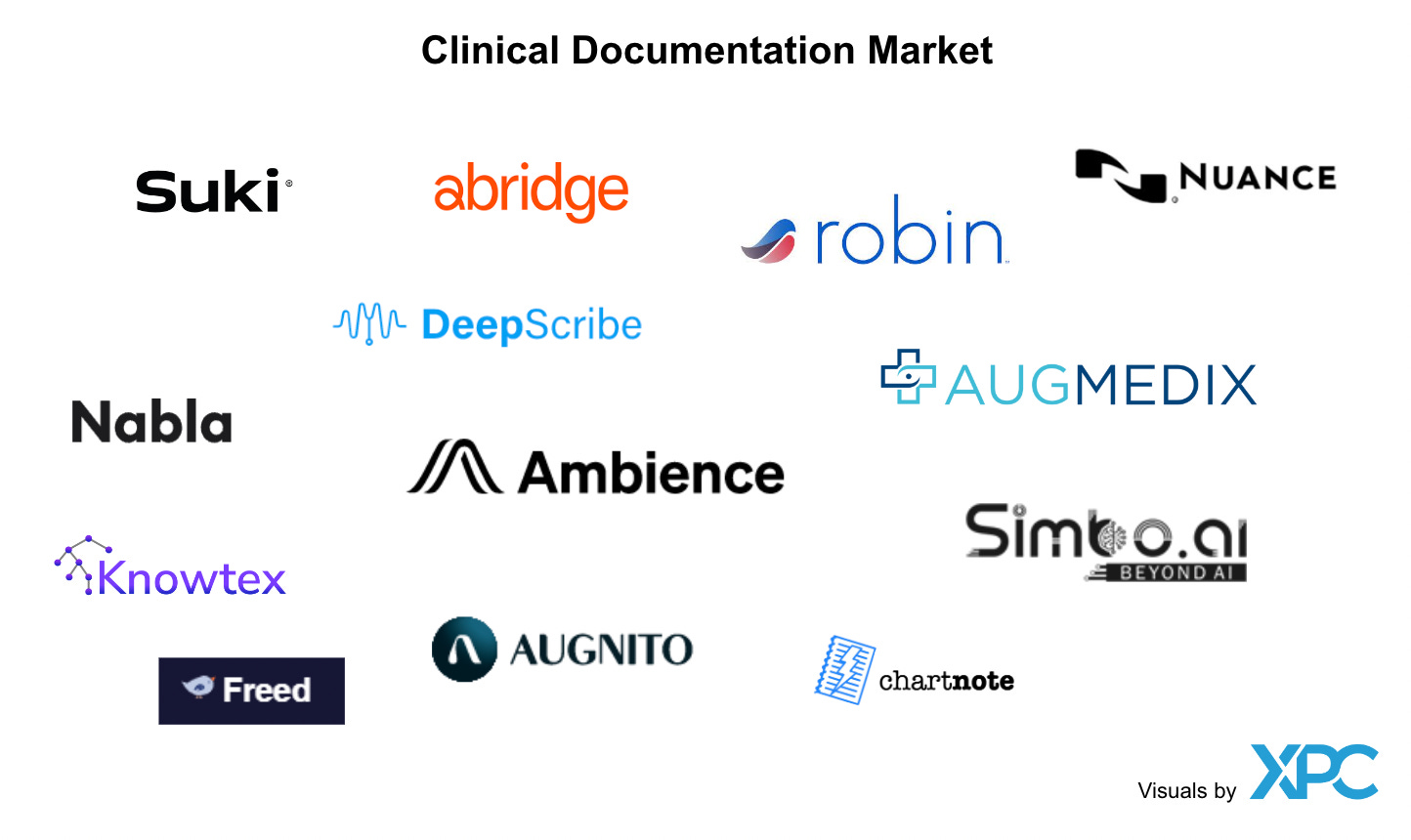
Diving into Ambient Documentation Economics and Financials
As Ben notes, ambient documentation solves a pressing need for health systems – whose relationships and contracts are extremely sticky.
Ambient documentation offers a sweet, sweet value prop for hospitals in 2023 when labor and margin pressures are top of mind:
- Quantifiable ROI to address clinician burnout (a $4.6B annual cost),
- A boost in clinician satisfaction – physicians love the product,
- An increase in patient engagement and medication adherence (hitting on consumerism trends and patient-facing communication/applications),
- Higher productivity (e.g., increase in visit volumes), and
- Optimized documentation for billing and EHR purposes.
For example, Augmedix (which is a public company and has data available) estimates that its solution saves doctors 2-3 hours a day.
Health system CFOs can do the math here. Between boosted physician productivity and burnout prevention/retention, seems like a pretty clear win-win-win for patient-provider-admin.
Just ask HCA Healthcare, the largest health system in the US that announced a partnership and investment in Augmedix today (4/20), providing a $12M capital infusion into the ambient player and giving the entire space even more investment credibility.
The pilot with HCA in two EDs will help Augmedix optimize the product for acute care settings. From the release:
Schlosser adds the pilot testing is an example of HCA Healthcare’s strategy of working closely with clinicians to design and integrate innovative technology into patient care. “This Augmedix proof-of-concept is not a complete solution, which is by design. We are capturing direct feedback from and ongoing collaboration with physicians and nurses to mold and develop the solution to meet their needs.” During the pilot, HCA Healthcare plans to gather data – including critical physician, nurse, and patient feedback – to determine the potential for use at additional HCA Healthcare hospitals.
So, it’s pretty easy to see why we’ve seen so much fundraising activity and new players enter the AI-enabled clinical workflow space. Some recent, non-exhaustive examples, total funding included:
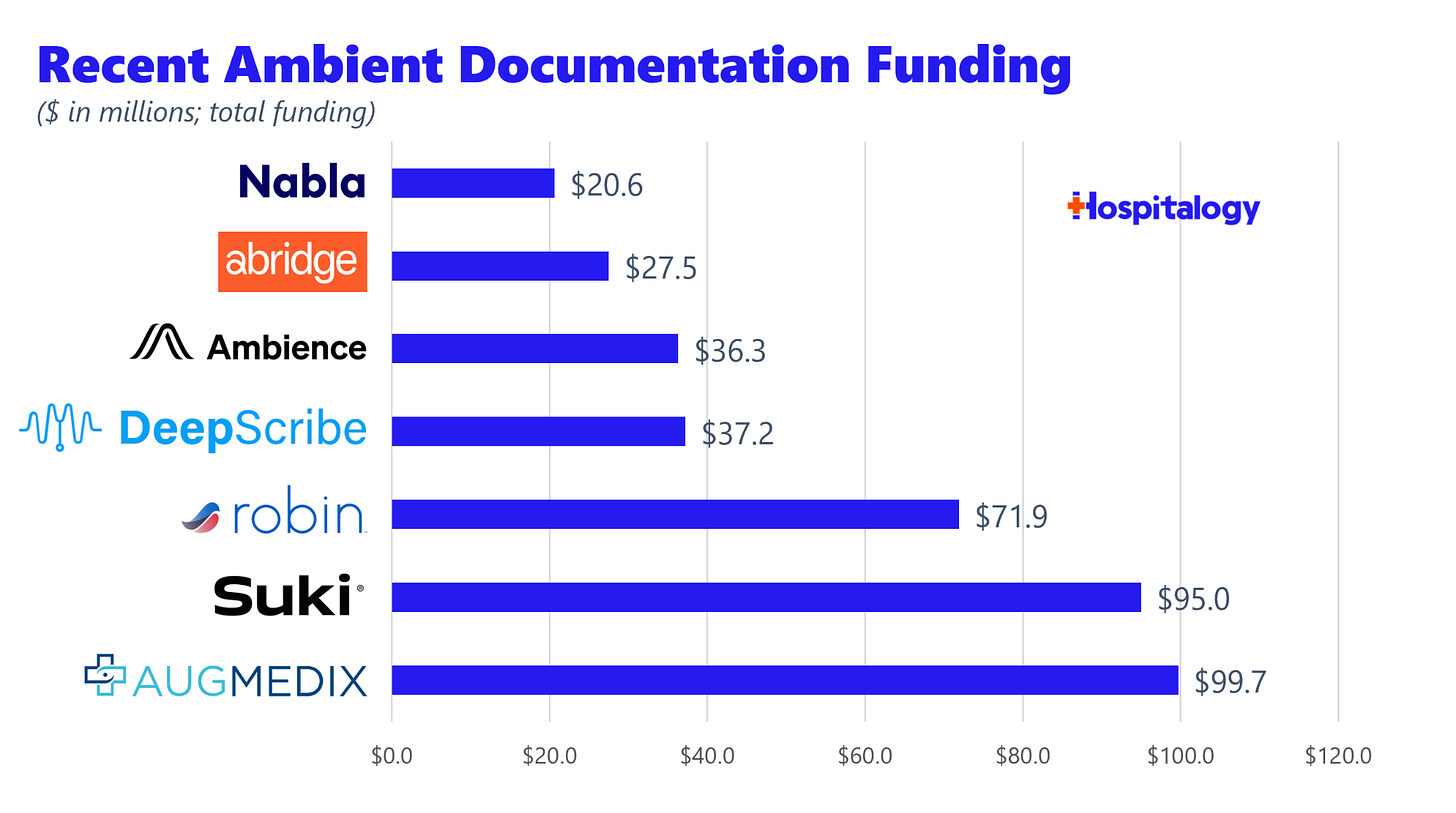
As far as the financial and business case, investors should like and pay attention to the AI scribing space. It’s one of the few areas in healthcare with a more scalable offering and SaaS-esque margins to boot.
Over time, the investment thesis Augmedix is presenting as a public company is intact. Its gross margin since 2019 has expanded, implying scalability. Moving forward, when vetting these companies financially, take a look at gross margins as a proxy for automation and operating leverage, cash burn, and ARR growth as a proxy for clinician growth:
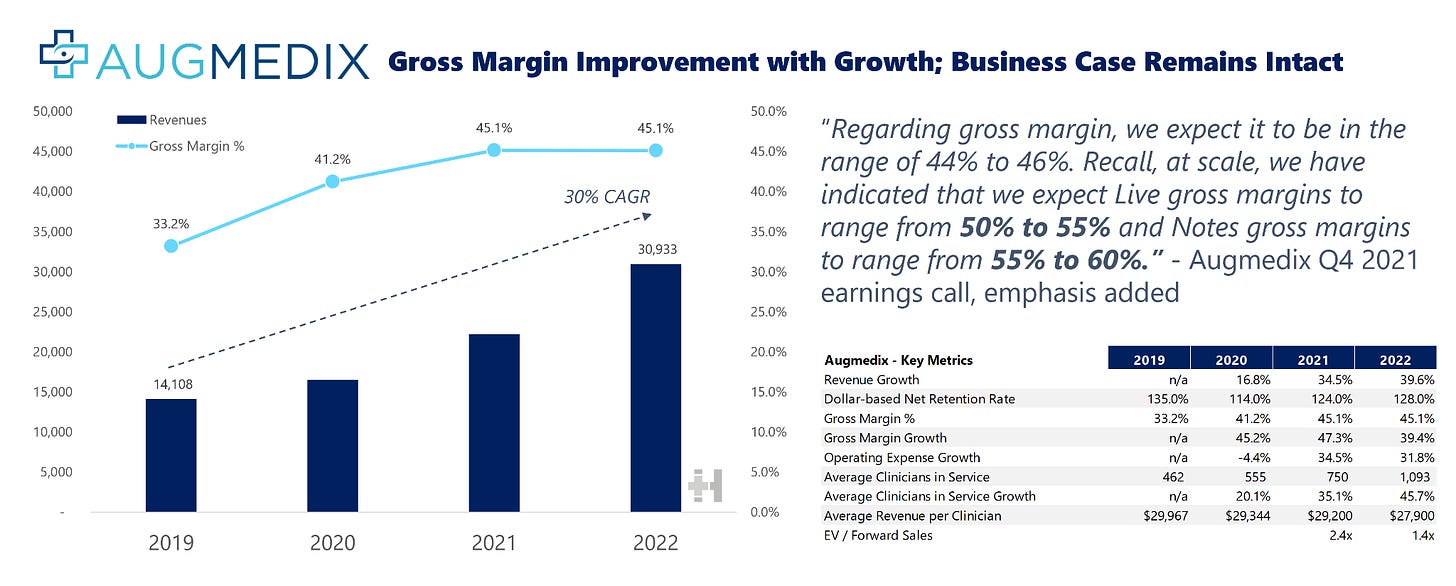
There’s a clear distinction here between players who provide hybrid offerings – with trained human medical scribes – versus pure-play, automated AI offerings.
Keep in mind that Augmedix operates the full spectrum of products here and its main ones, Notes and Live, still have a human component involved – its trained overseas medical scribe teams.
If new, fully automated products are actually worth a sniff (and without being a technical expert they seem to be trending in that direction), then gross margins would look rosier. I imagine that point is plastered in the decks of Ambience and Abridge, attracting the likes of Optum, a16z, OpenAI, Bessemer, Martin Ventures and other notables. The demo’s of new AI solutions are amazing. Like magic.
As these new automated offerings come online, it’ll be interesting to see whether they (1) truly are pure AI, automated and if so, (2) whether they cannibalize the current hybrid business models that have dominated the space recently (given that pricing is much lower for pure automated solutions).
As noted earlier in this piece, Augmedix thinks there’s still work to be done on the pure AI angle:
LLMs are particularly useful in providing rapid summaries and context from transcripts. However, use of LLMs alone today cannot produce medical notes that meet the [ accuracy ] standards or data structure requirements of our industry. A summary of the physician-patient encounter in the same sequence that the conversation unfolds and which contains some of the inconsistencies that invariably crop up in such conversations is not what the industry wants or needs. That is why we use other technologies together with LLMs to deliver highly accurate and structured medical notes and is a major reason why our note quality is considered so high by our customers. – Augmedix Q4 2022 earnings call, emphasis added
Kinda spicy take! It makes sense why the firm would make these comments given the highly specialized, trained medical scribes it employs in Bangladesh and other areas. To add to this, based on conversations I’ve had, in 2023, a pure AI solution will still require some form of human clean up. That gives the firms with the trained human medical scribe base the edge.
For now, everything I’ve seen points to AI as an augmenting tool rather than a full-fledged human replacement. Given the rate of change with AI lately though, I might be eating these words in like…a week.
Pure AI players will argue that the space is headed in this direction – that they’re more flexible, can compete on price, and differentiate by doubling down on AI-driven models. This angle makes sense – even Nuance and Augmedix are releasing and/or will release fully automated, software only products (DAX Express and Augmedix Go).
How Health Systems Vet Documentation Solutions
Given the human component involved, though, health systems are more likely to view existing hybrid solutions (Augmedix, Nuance) with a better sense of familiarity and comfortability. This dynamic is why Nuance and Augmedix dominate the enterprise space.
Leaders at health systems know how human medical scribes work. They don’t yet know how an AI-driven solution might fit in. It’s risky, and we all know how slow-moving these institutions are. Will it create compliance issues? Security issues? Is my hospital’s data at risk? All of these are likely addressable, but are additional hoops that AI-forward companies need to consider and deal with.
Here are some other considerations for documentation solutions from a health system perspective:
- Winning the contract is one thing; clinician change management is another. Physicians need to use these fantastic, time-saving tools – but many MDs are set in their ways and set aside time for documentation. Plus, lots of physicians prefer to work with human scribes. Perhaps there are ways to incentivize physicians to use new documentation tools, but adoption could prove a challenge.
- If the documentation is wrong or the code is wrong, then a human will need to spend time to fix it. Poor capturing could lead to a higher increase in denials from payors, which is a big issue.
- If these tools make clinicians 20%+ more efficient with their time, how will that truly translate in day to day clinical time? If physicians see even MORE patients in a day, could that still lead to productivity burnout? Health systems love the idea (and get sold on) of higher productivity and more visits in a day, but likely still need to tread carefully.
- Many health systems already view their employed medical scribes as a sunk cost – they have them, they trained them, and they might not want to get rid of them.
- In general, whether valid or not, generative AI creates a perceived security concern for hospital legal & compliance teams.
Inside the Health System bidding wars
As far as actual partnerships penned, the health system arms race is heating up. Like I noted, Nuance and Augmedix tend to be the two heavyweights when it comes to enterprise solutions, with some recent activity from Abridge:
- Nuance partnered with several large health systems recently including Cooper University Health Care, Providence, SCL Health, and WellSpan among others. Nuance also holds the ‘brand’ edge given its affiliation with Microsoft, OpenAI, and ChatGPT. Anecdotally, I hear it’s also the highest-priced vendor.
- Augmedix works with health systems including Sutter, Dignity, and UCSF and now HCA.
- Abridge recently won a partnership with The University of Kansas Health System
Health systems are looking for two primary things, which echoes HCA’s Sam Hazen’s commentary from my ViVE post – does the solution provide clear ROI to my organization? Show me the money. And…is this solution scalable across my entire organization? How does it integrate across different hospitals, specialties, and departments?
Pay attention to healthcare cloud dynamics and the major players trying to sell into health systems as well. They want to create compelling product offerings with the potential to cross-sell into health system clients.
- Azure is aligned with Microsoft-owned Nuance.
- Augmedix holds a close relationship with Google.
- Announced yesterday (yes, we have amazing timing), Amazon and AWS are now working with 3M’s M*Modal.
I’ve heard from industry personnel that Nuance and Microsoft are bidding to win health system contracts by cross-selling cloud capabilities. I imagine this pricing strategy grows more common in order to enter those sticky health system relationships.
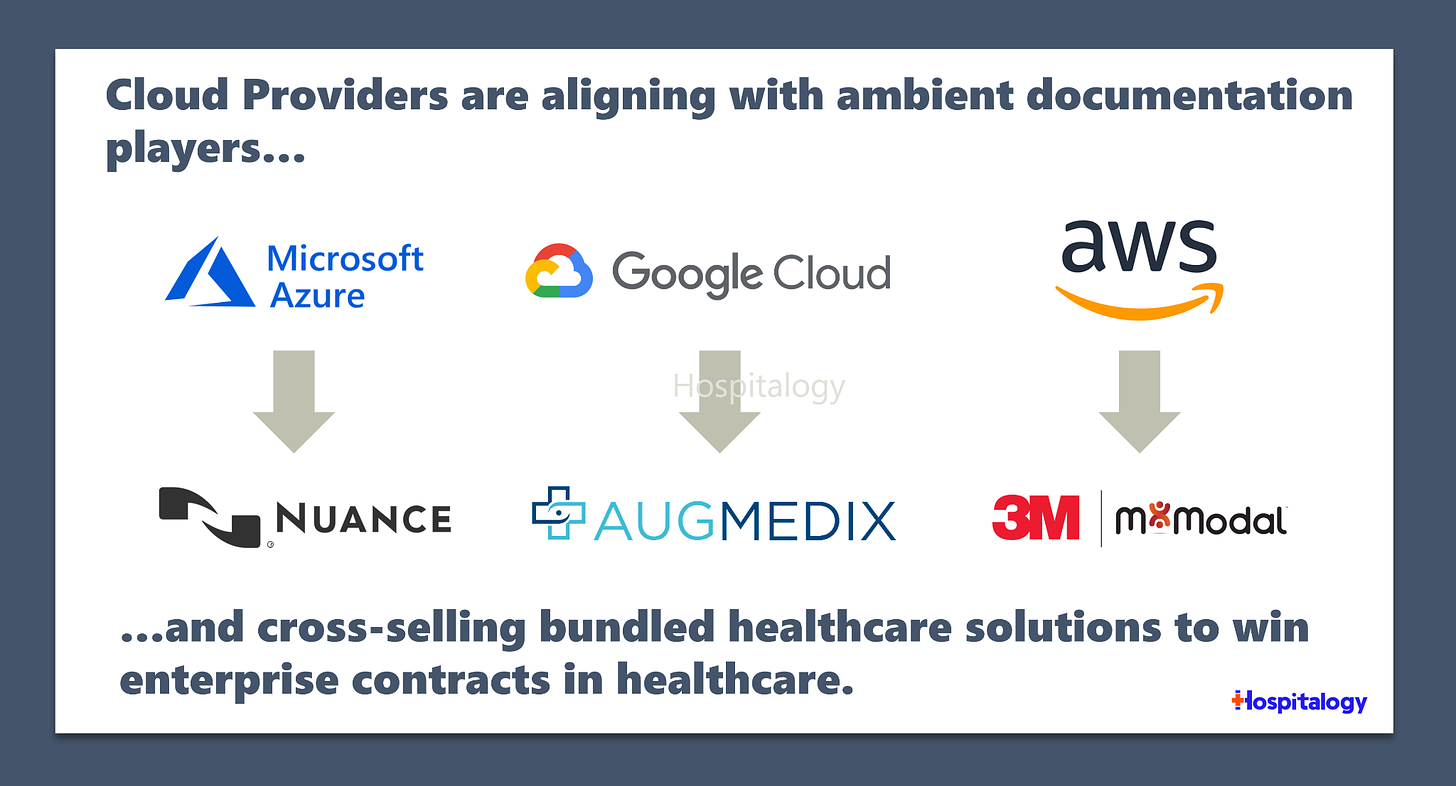
Parting thoughts from Blake
Documentation is an initial first wedge product into health systems. From there, there are plenty of other products and solutions to expand into, and I’ll be excited to see how AI-driven offerings change the game in the crusty ‘old hospital landscape. We’re in an exciting era of healthcare. It seems like the sky is the limit. Here’s hoping for thoughtful adoption of AI tools and strategies throughout health systems and enterprises.
If you have any thoughts on any of the assertions written above, feel free to give me a shout by replying to this newsletter! Find me here: [email protected]
Join 21,000+ executives and investors from leading healthcare organizations including VillageMD, Privia, and HCA Healthcare, health systems including Providence, Ascension, and Atrium, as well as leading digital health firms like Cityblock, Oak Street Health, and Turquoise Health by subscribing here!
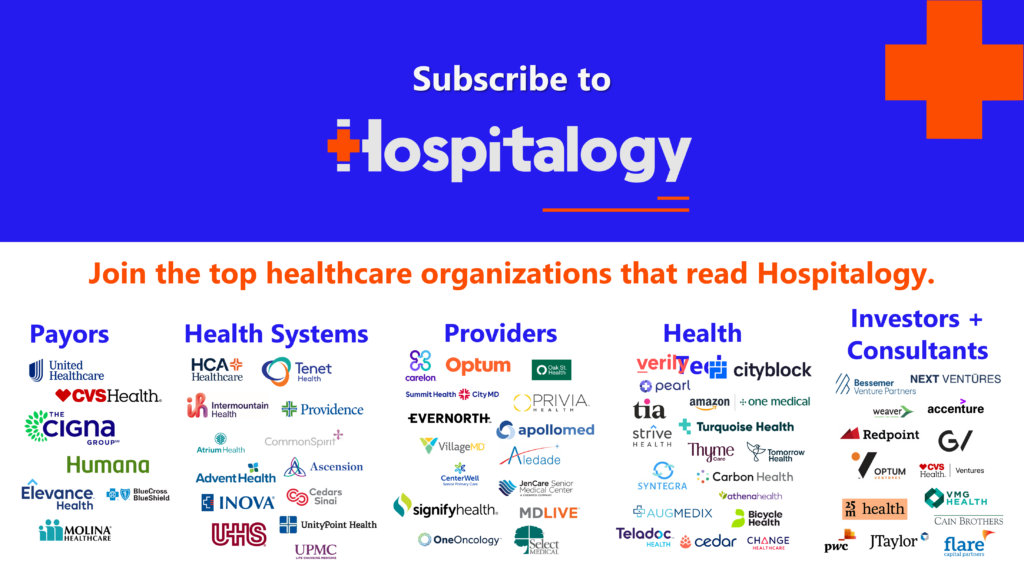